Machine learning may help in predicting likely rate of progression
Study into factors grouping Huntington's patients by disease progression rate
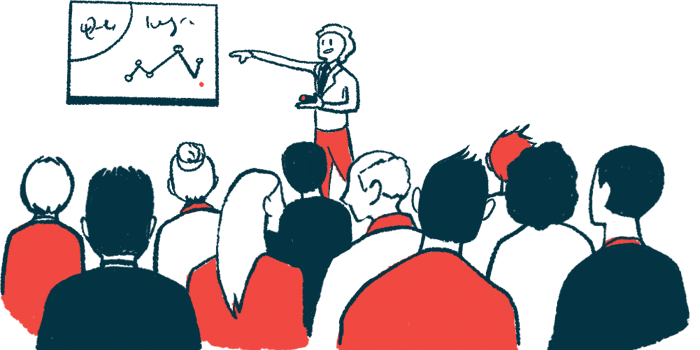
Using machine learning, a form of artificial intelligence, researchers were able to cluster people with Huntington’s disease into three groups based on the speed at which the disease progresses, a study showed.
“Machine learning approaches such as this could be considered for application in real-world clinical practice to support the treating physician in assessing whether patients are improving or worsening,” the researchers wrote.
They also noted that this approach could also be useful in clinical trials to assess whether experimental treatments are providing a benefit to patients.
The study, “Clustering and prediction of disease progression trajectories in Huntington’s disease: An analysis of Enroll-HD data using a machine learning approach,” was published in Frontiers in Neurology.
CAG repeat number, age, and disease duration seen to influence progression rate
Huntington’s is a progressive neurodegenerative disease caused by a mutation in the HTT gene called a CAG trinucleotide expansion, where three nucleotides (the building blocks of DNA) are repeated an excessive number of times.
The rate of Huntington’s progression and disease symptom profiles can vary considerably from person to person.
A team lead by researchers at Roche — which is developing tominersen as a potential therapy for Huntington’s — used machine learning to identify factors that might better predict how Huntington’s will progress.
Machine learning uses algorithms to analyze data, learn from its analyses, and then make a prediction about something.
For their analysis, the researchers retrieved data from Enroll-HD (NCT01574053), the world’s largest observational study of Huntington’s that is collecting information on the disease’s natural course from more than 20,000 people worldwide. Sponsored by the CHDI Foundation, the study is still recruiting participants at some 180 sites.
The analysis included data for 4,961 people with manifest Huntington’s, meaning they had begun to experience hallmark motor symptoms of the disease, and they had at least two annual visits on Enroll-HD.
Data on standardized measures covering a range of disease symptoms, including motor problems, cognitive difficulties, and mood disorders, were included.
“We selected … a set of clinically meaningful motor, cognitive and behavioral outcomes that clinicians were likely to be familiar with in clinical practice, and we showed the joint evolution of these outcomes in multiple dimensions,” the researchers wrote.
This information was fed into a computer, and the researchers conducted a clustering analysis — basically, giving the computer a set of mathematical instructions that it could use to sort the patients.
The data were based on the total motor score, which assesses motor function, the Symbol Digit Modalities Test that evaluates cognitive function, and the Problem Behaviors Assessment Short Form-Apathy.
“Clustering and prediction provide a profile for different rates of manifest HD [Huntington’s disease] progression, which may be useful to guide personalized clinical care and management plans,” the team wrote.
Results revealed three main clusters: about 29.2% of patients were slow progressors, 45.5% were moderate progressors, and 25.3% were rapid progressors. People in these three clusters were similar in terms of age at symptom onset and sex.
The scientists then looked for factors that could be used to predict which cluster a patient would be in.
They found that the CAP score at enrollment was the strongest predictors of rapid disease progression. The CAP score is a measure derived from a formula that accounts for CAG repeat number and a person’s age, and that results in higher scores with older age and longer CAG repeats.
Other strong predictors of rapid disease progression were disease duration and the absence of apathy, a common behavioral symptom of Huntington’s characterized by a lack of interest or concern about things.
Patients in the rapidly progressing cluster had moderate CAP scores, shorter disease duration, were less affected by apathy, had the lowest lower body mass index (a ratio of body weight to height), and were older at enrollment.
In turn, slow progressors showed lower CAP scores, longer disease duration, a history of apathy, higher BMI scores, and a younger age at enrollment visit. Patients with moderate disease progression had the highest CAP scores, moderate disease duration, a higher rate of apathy, higher BMI scores, and were older at enrollment.
“Knowledge of predictive features of manifest HD progression could guide the development of individualized clinical care and disease management approaches,” the researchers wrote.
The researchers noted that this approach could be used to analyze people with premanifest Huntington’s — individuals who carry a Huntington’s-causing mutation but have not begun to experience overt motor symptoms.
They also noted that adding more types of data beyond what was available from Enroll-HD might improve the accuracy of the models.