Artificial Intelligence Can ‘See’ Progression of Ills Like Huntington’s in Blood Sample, Study Says
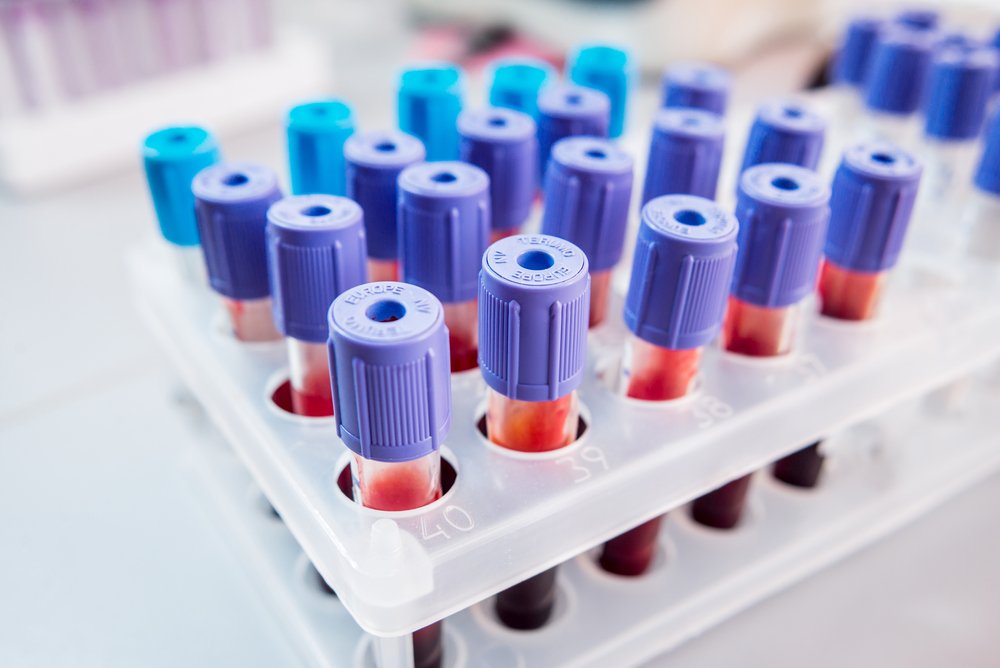
Artificial intelligence (AI) can predict disease progression using blood samples, a first long-term study of the molecular changes underlying neurodegeneration reports.
Scientists at McGill University trained an AI algorithm to analyze blood and post-mortem brain tissue from 1,969 people with Alzheimer’s and Huntington’s disease. From the blood samples, the algorithm was able to detect 80% of the same disease-related molecular pathways found in the brain samples.
It is hoped that the striking similarity between molecular alterations in the brain and blood imply that a simple blood test can soon be used to screen people for neurodegenerative diseases. It also raises the possibility of one day tailoring treatments to a patient’s unique genetic profile.
The study, “AI-analyzed blood test can predict the progression of neurodegenerative disease,” was published in the journal Brain.
Because of its highly invasive nature, gene expression studies of brain neurodegeneration necessarily rely on post-mortem tissue samples. Extrapolating post-mortem findings to the living is a major challenge.
To try and overcome this challenge, researchers compared the gene expression patterns in post-mortem brain samples with those of the blood of living patients at various disease stages. (Gene expression is the process by which information in a gene is synthesized to create a working product, like a protein.)
With access to decades worth of patient data, their algorithm was able to analyze how changes in these patients’ gene expression over time correlated with disease progression. The scientists hoped that this would reveal molecular patterns unique to these conditions.
Brain and blood samples were analyzed for patterns of gene expression at various disease stages, and compared to samples from healthy controls.
Individual gene expression closely tracked with disease features and progression. The genes found to be most influential to the disease states were involved in neurological and cognitive decay pathways.
Importantly, 85% and 90% of the disease-associated molecular pathways identified in two brain tissue datasets were also found to be most relevant in the blood samples. These common pathways, the researchers write, provide “evidence of the direct relationship between the CNS [central nervous system] and the body,” and support further study of this relationship.
Most previous research focusing on the gene activity patterns of neurodegeneration rely on “snapshots” of gene expression, taken at a single point in time. This study, in contrast, functioned more like a stop-motion sequence, arranging a series of snapshots into the illusion of a moving image.
It remains to be seen whether this new technique can be validated by replicating its results in further studies. Nonetheless, the team’s approach to studying disease progression has several benefits.
First, analyzing multiple tissue types is likely to prove useful in understanding the connections between both tissue-specific and systemic neurodegenerative mechanisms.
Second, interrogating dynamic changes in gene expression, rather than focusing on static time points, is vital for understanding the cellular transitions that take place over the course of a disease. This is especially important in diseases such as Huntington’s, which develops slowly over a long period of time.
Although this is not the first time that AI has been applied to Huntington’s research, it is one of very few studies to do so.
“This test could one day be used by doctors to evaluate patients and prescribe therapies tailored to their needs,” Yasser Iturria-Medina, the study’s first author, said in a McGill news release. “It could also be used in clinical trials to categorize patients and better determine how experimental drugs impact their predicted disease progression.”