Key Findings in Early Huntington’s Disease May Provide Clues for Research, Help Classify Puzzling Brain Disorders
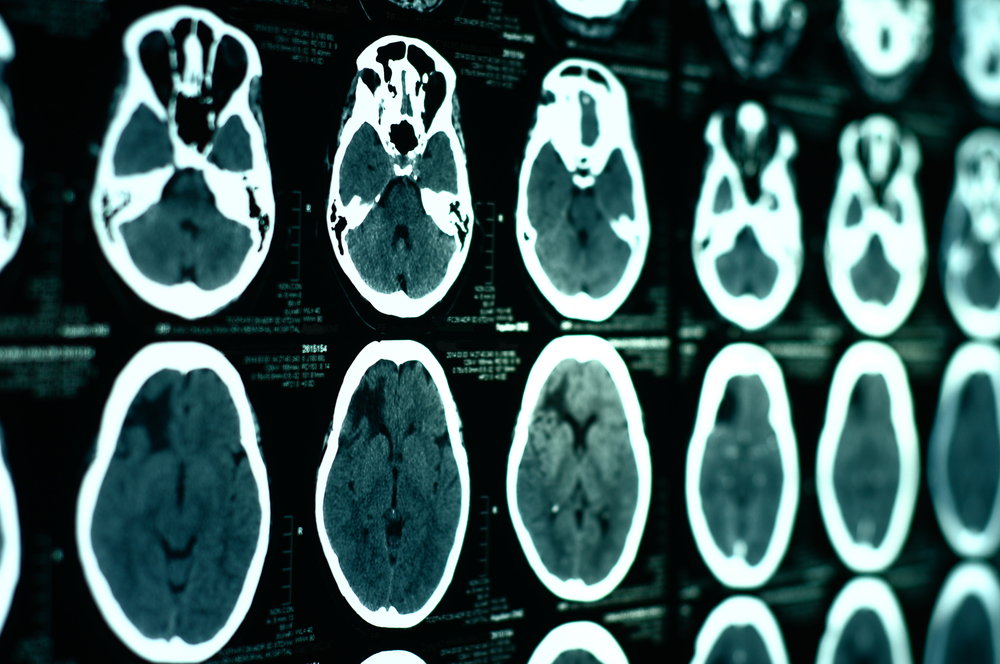
Researchers have developed a computational cognitive classification method that allows the differentiation of pre-symptomatic Huntington’s disease patients from early onset patients and healthy subjects, an approach that demonstrates promise for classification and prediction of brain disorders. The research paper, “A Computational Cognitive Biomarker for Early-Stage Huntington’s Disease,” was published in PLOS ONE.
Huntington’s disease, a neurodegenerative and debilitating condition, is caused by a known genetic mutation in the HTT gene. Despite knowledge of its cause, attempts at developing a drug that slows or reverses the disease have not been successful. Researchers say these failed attempts reveal a need for better clinical markers of the disease progression to give scientists a better understanding for an appropriate time for treatment initiation. This is a crucial factor in the success of treatment, researchers say.
Quantitative measures that are sensitive to early changes in pre-symptomatic patients could help researchers and clinicians establish the best times for therapeutic intervention.
Researchers wanted to establish quantitative predictors, through a computational approach and computational modeling, to predict Huntington’s disease before the onset of symptoms. The study included pre-symptomatic individuals (pre-HD), early symptomatic HD patients, and healthy controls.
The subjects performed behavioral testing through oculomotor and a series of other tasks, which involved executive control and response inhibition. Several different clinical measures were collected, including the standard assessment tool for HD symptom severity, the Unified Huntington’s Disease Rating Scale (UHDRS).
Results showed significant differences between symptomatic Huntington’s disease subjects and healthy controls in behavioral measures, such as reaction time. However, the same was not observed between controls and pre-HD subjects. When results were fit into a model that summarizes computational processes that relate to neuronal models, it was possible to identify key parameters related to executive control that differentiates pre-HD subjects from healthy controls.
In addition, the response inhibition parameter was observed to decline only after the onset of Huntington’s disease symptoms. Through this method of machine learning classification, scientists have demonstrated that the executive control parameter is predictive of Huntington’s disease prior to the onset of symptoms.
Despite acknowledging some limitations in the study, researchers concluded that their “findings demonstrate the utility of computational approaches for classification and prediction of brain disorders, and provide clues as to the underlying neural mechanisms.”